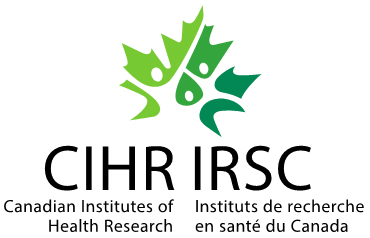
Aly Khalifa and Yuan Gao are awarded CIHR CGS-D fellowships for 3-years!
Unstructured datasets, including medical imaging, electrocardiograms, and natural language data, are gaining attention with advancements in deep convolutional neural networks and large language models. However, estimating the generalizability of these models to new healthcare settings without extensive validation on external data remains challenging. We propose an open source, bias-corrected external accuracy estimate that better estimates external accuracy to within 4% on average by measuring and calibrating for bias induced shortcut learning.
We describe an AI model that generates radiation treatment plans for prostate cancer patients. AI was successfully deployed in a standard-of-care clinical setting, providing gains in efficiency, improved treatment quality, and an understanding of physicians’ decision-making and perceptions of AI use when patient care is at stake.
Refinement of radiomic results and methodologies is required to ensure progression of the field. In this work, we establish a set of safeguards designed to improve and support current radiomic methodologies through detailed analysis of a radiomic signature.
We present a proof-of-concept for a method to automatically infer the radiation dose directly from the patient’s treatment planning image based on a database of previous patients with corresponding clinical treatment plans. Our method uses regression forests augmented with density estimation over the most informative features to learn an automatic atlas-selection metric that is tailored to dose prediction.
Aly Khalifa and Yuan Gao are awarded CIHR CGS-D fellowships for 3-years!
Sangwook Kim awarded DSI fellowship for 3-years!
Sangwook Kim successfully passes his PhD reclassification exam on Multi-Task Learning For Robust Deep Learning-Based Medical Image Analysis!
Yuan Gao successfully passes his PhD reclassification exam on Temporal Deep Learning Applications in Healthcare Monitoring and Wearables!
Aly Khalifa successfully passes his PhD reclassification exam on Automated Treatment Planning for Adaptive Radiation Therapy!
Siham successfully passes her PhD reclassification exam on Deep learning for Automatic Medical Image Segmentation!
Paper on prospective cancer radiotherapy using our AI platform published in Nature Medicine, including cover feature.
Cathy successfully passes her PhD reclassification exam on Developing an AI Image-Based Biomarker for Interventional Cardiovascular Risk-Stratification!
Drs. Rubin, Wong, and McIntosh featured in UHN foundation interview on Intelligent Outcomes in medicine with AI. Illustrations by Kyle Scott.
Paper on mass spectrometry gradient analysis of cancer to appear in Analytical Chemistry.
Dr. Chris McIntosh appointed as Chair in Medical Imaging and Artificial Intelligence at the Joint Department of Medical Imaging and University of Toronto.
Apple announces key partnership with Drs. Heather Ross, Yas Moayedi, and our team building AI with Apple Watch to support key clinical insights in heart failure.
Paper on cross domain fusion for MRI reconstruction accepted to MICCAI.
McIntosh Lab secures PMCC Innovation Award for proposal of an AI-driven system for automatic coronary angiographic interpretation.
Siham Amara-Belgadi (PhD Student)
William Gao (PhD Student)
Sangwook Kim (PhD Student)
Sejin Kim (PhD Student)
Cathy Ongly (PhD Student)
Balagopal Unnikrishnan (PhD Student)
Aly Khalifa (PhD Student)
Innovation doesn't happen in a vacuum. Jointly embedded in Canada's largest hospital network and the University of Toronto, our research brings together experts and trainees in computer science, medicine, biology, and physics to solve impactful problems at the intersection of artificial intelligence and medicine.
Medical domains: Our work includes a variety of data modalities (biological, wearable, structured reporting, and imaging) and critical diseases such as cancer and heart disease. We work closely with clinicians to understand and ultimately augment patient care with AI.
AI technologies: Solving clinical problems means pushing and extending the boundaries of AI technology including semi-supervised learning, domain adaptation/model generalization, meta-learning, and multi-modal data fusion.
Data: We work on datasets of all shapes and sizes, from rare diseases with rich structured data but few patients, to diverse imaging datasets in the tens of thousands.
Keep reading below for example projects.
Wearable technologies offer a unique glimpse into patient function and biology outside of episodes of care. If AI is the present, wearbles with AI are the next frontier. Working with leading cardiologists Heather Ross and Yas Moyaedi, the Ted Rogers Centre for Heart Research, and a key partnership with Apple we are developing AI technology to asses the role of wearbles in heart failure.
Multimodal large language models in healthcare represent a cutting-edge fusion of advanced linguistic capabilities and diverse data sources. By seamlessly integrating text, images, electrocardiograms, and more these models harbor great potential to enhance clinical care through more robust and generalizable machine learning.
An experienced clinical team can more than double a cancer patient’s odds of survival. In particular, radiation therapy (RT) is a key part of the treatment for 40% of all cancer patients with the potential to be highly curative and effective given the right treatment plan. However, a major barrier in that effectiveness is the expertise and experience of the patient’s clinical team in his or her particular cancer. We believe that AI can help bring the expertise of the world’s best clinical teams to every patient in every hospital, saving lives and costly resources.
Working with clinical partners (Tom Purdie, Ale Berlin, and Leigh Conroy) we have developed the world’s first computer vision treatment system that we piloted to treat prostate cancer patients at Princess Margaret Cancer Centre, with superior results to our standard of care for the majority of patients. Cancer treatments are generated by the AI in minutes, instead of the hours-to-days taken under the typical manual process, increasing both efficiency and quality of care. Follow-up studies are expanding the models to breast cancer, brain tumours in young adults, and more. Through a successful licensing deal of this Canadian technology to RaySearch Laboratories we are also supporting deployment across Canada and around the world.
Image segmentation is the gold standard for computational morphology and a key step in the radiation therapy process. A long sought after technology, automated segmentation methods are recently achieving near clinical performance paving the way for clinical studies, but many challenges remain.
With global acquisition rates of medical imaging ever increasing, imaging represents a significant resource of potential prognostic value. Using combinations of deep learning and computer vision technologies to extract quantifiable features from imaging to risk stratify patients and better understand their care is growing area of research.
"Jobs Help Wanted" by Innov8social is licensed under CC BY 2.0
We are seeking a postdoctoral fellow with experience in medicine and AI. Expertise is required in machine learning and programming in the context of at least one of medical signals, imaging, or wearable data.
Interested students can submit a CV, a copy of your most relevant publication, and the names and contact information for up to three references to Chris McIntosh, with the subject line Postdoctoral application.
We are seeking motivated MSc/PhD students with a keen interest in medicine and AI. Expertise in at least one topic (machine learning, programming, and biology) and experience in others is desired.
Interested students must apply to either the Department of Medical Biophysics (preferred), or Computer Science as appropriate to their interests and backgrounds.
We welcome undergraduate internships/summer students with a keen interest in medicine and AI. Experience in at least one topic (machine learning, programming, and biology) is required.
Interested students are encouraged to apply to the MBP summer student program.